Soluciones tecnológicas para el desarrollo de la agricultura de precisión a través de imágenes multiespectrales.
Keywords:
Soluciones, Tecnológicas, Desarrollo, Agricultura, Precisión, Imágenes, MultiespectralesSynopsis
En este libro los autores pretenden transmitir la información necesaria y los resultados de investigaciones que demuestren que, por medio de la implementación de las imágenes multiespectrales como herramienta de adquisición remota de información para el monitoreo de cultivos, se puede identificar de antemano las características del terreno en cuanto a la calidad y cantidad de la vegetación presente, además, de la identificación de problemas en las áreas que son materia de estudio.
Se busca destacar la integración del sistema en UAV, lo que permite, entre otras ventajas, una movilización rápida y eficiente por grandes extensiones de tierra a cualquier altura, complementando las actividades productivas de los cultivadores en el desarrollo de métodos de inspección no destructiva que permite suministrar información real y precisa de los cultivos de diferentes modelos agroecológicos.
Downloads
References
Akatsu, T., Takiguchi, Y., Shinoda, Y., Wakai, F., & Muto, H. (2022). Optical transmittance and electrical conductivity of silica glass with biserial and hierarchical network structures made of carbon nanofi-bers. Ceramics International, 48(24), 36515–36520. https://doi.org/10.1016/J.CERAMINT.2022.08.211
Alabi, T. R., Abebe, A. T., Chigeza, G., & Fowobaje, K. R. (2022). Estimation of soybean grain yield from multispectral high-resolution UAV data with machine learning models in West Africa. Remote Sensing Applications: Society and Environment, 27, 100782. https://doi.org/10.1016/J.RSASE.2022.100782
Almeida, P. A. D., & Gregorio-Hetem, J. (2022). Aspectos do Sol observados em diferentes faixas espectrais. Revista Brasileira de Ensino de Física, 44, e202100405. https://doi.org/10.1590/1806-9126-rbef-2021-0405
Alvarenga, C. B., Mundim, G. S. M., Santos, E. A., Gallis, R. B. A., Zampiroli, R., Rinaldi, P. C. N., & Prado, J. R. (2023). Normalized difference vegetation index for desiccation evaluation with glyphosate 2,4-D in magnetized spray solution. Brazilian Journal of Biology, 83, e246579. https://doi.org/10.1590/1519-6984.246579
Atia, A., Bouabdallah, S., Ghernaout, B., Teggar, M., & Benchatti, T. (2023). Investigation of various absorber surface shapes for performance improvement of solar chimney power plant. Applied Thermal Engineering, 235, 121395. https://doi.org/10.1016/J.APPLTHERMALENG.2023.121395
Bastiaanssen, W. G. M., Molden, D. J., & Makin, I. W. (2000). Remote sensing for irrigated agriculture: examples from research and posible applications. Agricultural Water Manage research and possible applications. Agricultural Water Management, 46(2), 137–155. https://doi.org/https://doi.org/10.1016/S0378-3774(00)00080-9
Bendig, J., Malenovský, Z., Gautam, D., & Lucieer, A. (2020). Solar-In-duced Chlorophyll Fluorescence Measured From an Unmanned Aircraft System: Sensor Etaloning and Platform Motion Correction. IEEE Transactions on Geoscience and Remote Sensing, 58(5), 3437–3444. https://doi.org/10.1109/TGRS.2019.2956194
Bonnaire Rivera, L., Montoya Bonilla, B., & Obando-Vidal, F. (2021). Procesamiento de imágenes multiespectrales captadas con drones para evaluar el índice de vegetación de diferencia normalizada en plantaciones de café variedad Castillo. Ciencia & Tecnología Agropecuaria, 22(1). https://doi.org/10.21930/rcta.vol22_num1_art:1578
Bourgeon, M.-A., Paoli, J.-N., Jones, G., Villette, S., & Gée, C. (2016). Field radiometric calibration of a multispectral on-the-go sensor dedicated to the characterization of vineyard foliage. Computers and Electronics in Agriculture, 123, 184–194. https://doi.org/https://doi.org/10.1016/j.compag.2016.02.019
Bouvet, M. (2014). Radiometric comparison of multispectral imagers over a pseudo-invariant calibration site using a reference radiometric model. Remote Sensing of Environment, 140, 141–154. https://doi.org/10.1016/J.RSE.2013.08.039
Cao, S., Danielson, B., Clare, S., Koenig, S., Campos-Vargas, C., & Sanchez-Azofeifa, A. (2019). Radiometric calibration assessments for UAS-borne multispectral cameras: Laboratory and field protocols. ISPRS Journal of Photogrammetry and Remote Sensing, 149, 132–145. https://doi.org/https://doi.org/10.1016/j.isprsjprs.2019.01.016
Castro Sardiña, L., Irisarri, G., & Texeira, M. (2023). Climate factors rather than human activities controlled NDVI trends across wet meadow areas in the Andes Centrales of Argentina. Journal of Arid Environments, 214, 104983. https://doi.org/10.1016/J.JARIDENV.2023.104983
Chaminda Bandara, W. G., Kasun Prabhath, G. W., Sahan Chinthana Bandara Dissanayake, D. W., Herath, V. R., Roshan Indika Godaliyadda, G. M., Bandara Ekanayake, M. P., Demini, D., & Madhujith, T. (2020). Validation of multispectral imaging for the detection of selected adulterants in turmeric samples. Journal of Food Engineering, 266, 109700. https://doi.org/10.1016/J.JFOODENG.2019.109700
Cui, Z., Wang, Y., Gao, X., Li, J., & Zheng, Y. (2016). Multispectral image classification based on improved weighted MRF Bayesian. Neurocomputing, 212, 75–87. https://doi.org/https://doi.org/10.1016/j.neucom.2016.03.097
Davidson, C., Jaganathan, V., Sivakumar, A. N., Czarnecki, J. M. P., & Chowdhary, G. (2022). NDVI/NDRE prediction from standard RGB aerial imagery using deep learning. Computers and Electronics in Agriculture, 203, 107396. https://doi.org/10.1016/J.COMPAG.2022.107396
Davis, Z., Nesbitt, L., Guhn, M., & van den Bosch, M. (2023). Assessing changes in urban vegetation using Normalised Difference Vegetation Index (NDVI) for epidemiological studies. Urban Forestry & Urban Greening, 88, 128080. https://doi.org/10.1016/J.UFUG.2023.128080
De Abreu Fontes, J., Anzanello, M. J., Brito, J. B. G., Bucco, G. B., Fogliatto, F. S., & Puglia, F. do P. (2021). Combining wavelength importance ranking to the random forest classifier to analyze multiclass spectral data. Forensic Science International, 328, 110998. https://doi.org/10.1016/J.FORSCIINT.2021.110998
Deng, L., Mao, Z., Li, X., Hu, Z., Duan, F., & Yan, Y. (2018). UAV-based multispectral remote sensing for precision agriculture: A comparison between different cameras. ISPRS Journal of Photogrammetry and Remote Sensing, 146, 124–136. https://doi.org/https://doi.org/10.1016/j.isprsjprs.2018.09.008
Doering, D., Vizzotto, M. R., Bredemeier, C., da Costa, C. M., Henriques, R. V. B., Pignaton, E., & Pereira, C. E. (2016). MDE-based Development of a Multispectral Camera for Precision Agriculture. IFAC-PapersOnLine, 49(30), 24–29. https://doi.org/https://doi.org/10.1016/j.ifacol.2016.11.117
Duan, T., Chapman, S. C., Guo, Y., & Zheng, B. (2017). Dynamic monitoring of NDVI in wheat agronomy and breeding trials using an unmanned aerial vehicle. Field Crops Research, 210, 71–80. https://doi.org/https://doi.org/10.1016/j.fcr.2017.05.025
González Bosquet, L. (2003). Los efectos nocivos de la radiación solar y la forma de combatirlos. Offarm, 22(5), 68–76. https://www.elsevier.es/es-revista-offarm-4-articulo-los-efectos-nocivos-radiacion-solar-13047747
Gregg, J. F., Anderson, B. E., & Remillieux, M. C. (2020). Electromagnetic excitation technique for nonlinear resonant ultrasound spectroscopy. NDT & E International, 109, 102181. https://doi.org/10.1016/J.NDTEINT.2019.102181
Hoffmann, H.-J. (2001). Optical Glasses. Encyclopedia of Materials: Science and Technology, 6426–6441. https://doi.org/10.1016/B0-08-043152-6/01137-2
Invernizzi, D., & Lovera, M. (2018). Trajectory tracking control of thrust-vectoring UAVs. Automatica, 95, 180–186. https://doi.org/https://doi.org/10.1016/j.automatica.2018.05.024
Ivushkin, K., Bartholomeus, H., Bregt, A. K., Pulatov, A., Franceschini, M. H. D., Kramer, H., van Loo, E. N., Roman, V. J., & Finkers, R. (2019). UAV based soil salinity assessment of cropland. Geoderma, 338, 502–512. https://doi.org/https://doi.org/10.1016/j.geoderma.2018.09.046
Kaljahi, M. A., Shivakumara, P., Idris, M. Y. I., Anisi, M. H., Lu, T., Blumenstein, M., & Noor, N. M. (2019). An automatic zone detection system for safe landing of UAVs. Expert Systems with Applications, 122, 319–333. https://doi.org/https://doi.org/10.1016/j.eswa.2019.01.024
Lan, Y., Huang, Z., Deng, X., Zhu, Z., Huang, H., Zheng, Z., Lian, B., Zeng, G., & Tong, Z. (2020). Comparison of machine learning methods for citrus greening detection on UAV multispectral images. Computers and Electronics in Agriculture, 171, 105234. https://doi.org/https://doi.org/10.1016/j.compag.2020.105234
L’Annunziata, M. F. (2023). Electromagnetic Radiation: photons. Radioactivity, 709–746. https://doi.org/10.1016/B978-0-323-90440-7.00005-3
Maes, W. H., & Steppe, K. (2019). Perspectives for Remote Sensing with Unmanned Aerial Vehicles in Precision Agriculture. Trends in Plant Science, 24(2), 152–164. https://doi.org/https://doi.org/10.1016/j.tplants.2018.11.007
Maimaitijiang, M., Sagan, V., Sidike, P., Hartling, S., Esposito, F., & Fritschi, F. B. (2020). Soybean yield prediction from UAV using multimodal data fusion and deep learning. Remote Sensing of Environment, 237, 111599. https://doi.org/https://doi.org/10.1016/j.rse.2019.111599
Mikhail, I. E., Hemida, M., Lebanov, L., Astrakhantseva, S., Gupta, V., Hortin, P., Parry, J. S., Macka, M., & Paull, B. (2023). Multi-wavelength deep-ultraviolet absorbance detector based upon program-controlled pulsing light-emitting diodes. Journal of Chromatography A, 1709, 464382. https://doi.org/10.1016/J.CHROMA.2023.464382
Nanda Kumar, K., Vijayan Pillai, A., & Badri Narayanan, M. K. (2021). Smart agriculture using IoT. Materials Today: Proceedings. https://doi.org/https://doi.org/10.1016/j.matpr.2021.02.474
Nandibewoor, A., Hebbal, S. B., & Hegadi, R. (2015). Remote Monitoring of Maize Crop through Satellite Multispectral Imagery. Procedia Computer Science, 45, 344–353. https://doi.org/https://doi.org/10.1016/j.procs.2015.03.158
Orlando, S., Minacapilli, M., Sarno, M., Carrubba, A., & Motisi, A. (2022). A low-cost multispectral imaging system for the characterisation of soil and small vegetation properties using visible and near-infra-red reflectance. Computers and Electronics in Agriculture, 202, 107359. https://doi.org/10.1016/J.COMPAG.2022.107359
Pelaez Carrillo, D. A., & Gualdron Guerrero, O. E. (2020). Caracterización de suelos con potencial productivo en el departamento de Norte de Santander usando cámara multiespectral en un vehículo aéreo no tripulado [Universidad de Pamplona]. http://repositoriodspace.unipamplona.edu.co/jspui/handle/20.500.12744/3330
Pelaez, D. A., Gualdron, O. E., & Castellanos, L. (2020). Analysis of the intensity of electromagnetic radiation for the estimation of vegetation cover. Journal of Physics: Conference Series, 1704, 12008. https://doi.org/10.1088/1742-6596/1704/1/012008
Pelaez, D. A., Gualdron, O. E., & Torres, I. (2020). Soil characterization through remote acquisition of electromagnetic radiation. Journal of Physics: Conference Series, 1587, 12033. https://doi.org/10.1088/1742-6596/1587/1/012033
Pu, H., Kamruzzaman, M., & Sun, D.-W. (2015). Selection of feature wavelengths for developing multispectral imaging systems for quality, safety and authenticity of muscle foods-a review. Trends in Food Science & Technology, 45(1), 86–104. https://doi.org/https://doi.org/10.1016/j.tifs.2015.05.006
Rahiche, A., Hedjam, R., Al-maadeed, S., & Cheriet, M. (2020). Historical documents dating using multispectral imaging and ordinal classification. Journal of Cultural Heritage, 45, 71–80. https://doi.org/10.1016/J.CULHER.2020.01.012
Rodríguez, J. M. (2018). Polarización de la luz: conceptos básicos y aplicaciones en astrofísica. Revista Brasileira de Ensino de Física, 40(4), e4310. https://doi.org/10.1590/1806-9126-rbef-2018-0024
Ruiz-Arias, J. A. (2022). Spectral integration of clear-sky atmospheric transmittance: Review and worldwide performance. Renewable and Sustainable Energy Reviews, 161, 112302. https://doi.org/10.1016/J.RSER.2022.112302
Shafiee, S., Mroz, T., Burud, I., & Lillemo, M. (2023). Evaluation of UAV multispectral cameras for yield and biomass prediction in wheat under different sun elevation angles and phenological stages. Computers and Electronics in Agriculture, 210, 107874. https://doi.org/10.1016/J.COMPAG.2023.107874
Singh, P. J., & Silva, R. de. (2018). Design and implementation of an experimental UAV network. 2018 International Conference on Information and Communications Technology (ICOIACT), 168–173. https://doi.org/10.1109/ICOIACT.2018.8350739
Sun, B., Bi, L., Yang, P., Kahnert, M., & Kattawar, G. (2020). Fundamentals. Invariant Imbedding T-Matrix Method for Light Scattering by Nonspherical and Inhomogeneous Particles, 7–56. https://doi.org/10.1016/B978-0-12-818090-7.00002-4
Suomalainen, J., Oliveira, R. A., Hakala, T., Koivumäki, N., Markelin, L., Näsi, R., & Honkavaara, E. (2021). Direct reflectance transformation methodology for drone-based hyperspectral imaging. Remote Sensing of Environment, 266, 112691. https://doi.org/10.1016/J.RSE.2021.112691
Talaviya, T., Shah, D., Patel, N., Yagnik, H., & Shah, M. (2020). Implementation of artificial intelligence in agriculture for optimisation of irrigation and application of pesticides and herbicides. Artificial Intelligence in Agriculture, 4, 58–73. https://doi.org/https://doi.org/10.1016/j.aiia.2020.04.002
Verrelst, J., Rivera, J. P., Gitelson, A., Delegido, J., Moreno, J., & Camps-Valls, G. (2016). Spectral band selection for vegetation properties retrieval using Gaussian processes regression. International Journal of Applied Earth Observation and Geoinformation, 52, 554–567. https://doi.org/https://doi.org/10.1016/j.jag.2016.07.016
Walshe, D., McInerney, D., Kerchove, R. Van De, Goyens, C., Balaji, P., & Byrne, K. A. (2020). Detecting nutrient deficiency in spruce forests using multispectral satellite imagery. International Journal of Applied Earth Observation and Geoinformation, 86, 101975. https://doi.org/https://doi.org/10.1016/j.jag.2019.101975
Wang, B., Ren, M., Xia, C., Li, Q., Dong, M., Zhang, C., Guo, C., Liu, W., & Pischler, O. (2022). Evaluation of insulator aging status based on multispectral imaging optimized by hyperspectral analysis. Measurement, 205, 112058. https://doi.org/10.1016/J.MEASUREMENT.2022.112058
Xu, L., Ming, D., Zhang, L., Dong, D., Qing, Y., Yang, J., & Zhou, C. (2023). Parcel level staple crop type identification based on newly defined red-edge vegetation indices and ORNN. Computers and Electronics in Agriculture, 211, 108012. https://doi.org/10.1016/J.COMPAG.2023.108012
Yavru, C. A., Kaleli, M., Üncü, İ. S., Koç, M., & Aldemir, D. A. (2022). Solar and infrared light sensing comparison of Yb/CIGS photodiode. Sensors and Actuators A: Physical, 347, 113973. https://doi.org/10.1016/J.SNA.2022.113973
Yu, Y., Shi, F., Zhang, Y., Li, F., & Han, J. (2024). Optical sensor array for the discrimination of liquors. Journal of Future Foods, 4(1), 48–60. https://doi.org/10.1016/J.JFUTFO.2023.05.004
Yue, J., & Tian, Q. (2020). Estimating fractional cover of crop, crop residue, and soil in cropland using broadband remote sensing data and machine learning. International Journal of Applied Earth Observation and Geoinformation, 89, 102089. https://doi.org/10.1016/J.JAG.2020.102089
Zhang, L., Shi, J., Zhu, Y., Zhang, C., Zhang, Z., & Zheng, J. (2023). An experimental study on monitoring wave profiles with LiDAR. Ocean Engineering, 285, 115436. https://doi.org/10.1016/J.OCEANENG.2023.115436
Zhang, T., Guan, H., Ma, X., & Shen, P. (2023). Drought recognition based on feature extraction of multispectral images for the soybean canopy. Ecological Informatics, 77, 102248. https://doi.org/10.1016/J.ECOINF.2023.102248
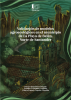
Published
Versions
Series
Categories
License

This work is licensed under a Creative Commons Attribution-NonCommercial 4.0 International License.